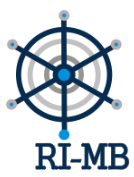
Use este identificador para citar ou linkar para este item:
https://www.repositorio.mar.mil.br/handle/ripcmb/846557
Título: | Avaliação de técnicas de Machine Learning na classificação de alvos marítimos em imagens SAR na Banda C |
Autor(es): | Silva, Fabiano Gabriel da |
Orientador(es): | Machado, Renato Palm, Bruna Gregory |
Palavras-chave: | SAR-ATR Machine Learning Deep Learning |
Áreas de conhecimento da DGPM: | Telecomunicações |
Data do documento: | 2022 |
Editor: | Instituto Tecnológico da Aeronáutica (ITA) |
Descrição: | Esta pesquisa faz uma avaliação da classificação de alvos em ambiente marítimo através de imagens de radar de abertura sintética - synthetic aperture radar (SAR), técnicas de machine learning (ML) e deep learning (DL). Os alvos de interesse são plataformas e navios localizados na bacia de Campos/RJ. Para identificação dos alvos, utiliza-se um banco de imagens SAR polarimétricas vertical-horizontal (VH) e vertical-vertical (VV) obtidas de sensores orbitais do sistema Sentinel-1. Duas redes neurais convolucionais - convolutional neural network (CNN), visual geometry group (VGG), VGG-16 e VGG- 19 são utilizadas para vetorização e extração de atributos. A classificação das imagens é dividida em seis formas distintas, considerando as seguintes técnicas de ML: logistic regression (LR), support vector machine (SVM), random forest (RF), k nearest neighbor (kNN), naive Bayes (NB), decision tree (DT), adaboost (ADBST) e neural network (NET). As amostras são geradas através da técnica bootstrap. Utiliza-se treinamento supervisionado, com 80% das amostras para treino e 20% das amostras para teste. A metodologia considera seis métodos de classificação. Para cada método são realizadas 50 classificações. Todos os classificadores foram avaliados em relação a CNN (VGG-16 e VGG-19) e a polarização (VH e VV). Os maiores níveis de acurácia são obtidos pelo LR com 85,5% e 86,5%. A técnica principal components analysis (PCA) aumenta a performance dos classificadores NB e RF. A combinação dos data sets permite aumentar a variabilidade das amostras de treinamento, proporcionando maior capacidade de generalização. A técnica stacking apresenta melhores resultados que as classificações individuais. Os índices de acurácia superiores a 80% concentram-se no canal de polarização VH e são distribuídos de forma equivalente entre as CNN VGG-16 e VGG-19. Por fim, os resultados foram comparados pelos testes estatísticos Kruskal-Wallis e Dunn que comprovam haver diferença significativa entre os métodos de classificação, ou seja, as otimizações (configurações de parâmetros e combinações de técnicas) proporcionam resultados competitivos com a literatura. Diante disso, a pesquisa contribui para ampliação dos métodos de vigilância da Amazônia Azul através de técnicas de inteligência artificial consideradas estado da arte. |
Abstract: | This research evaluates the classification of targets in a maritime environment through synthetic aperture radar (SAR) images, machine learning (ML), and deep learning (DL) techniques. The targets of interest are platforms and vessels located in the Campos/RJ basin. A data set of polarimetric vertical-horizontal (VH) and vertical-vertical (VV) SAR images obtained from orbital sensors of the Sentinel-1 system is used to identify the tar- gets. Two convolutional neural networks (CNN), visual geometry group (VGG), VGG-16, and VGG-19, are used for vectorization and feature extraction. The classification of ima- ges is divided into six different ways, considering the following ML techniques: logistic regression (LR), support vector machine (SVM), random forest (RF), k nearest neighbor (kNN), naive Bayes (NB), decision tree (DT), adaboost (ADBST), and neural network (NET). Samples are generated using the bootstrap technique. Supervised training is used, with 80% of samples for training and 20% of samples for testing. The methodology con- siders six classification methods. for each method 50 classifications are performed. All classifiers were evaluated about CNN (VGG-16 and VGG-19) and polarization (VH and VV). The LR obtains the highest levels of accuracy with 85.5% and 86.5%. The principal components analysis (PCA) technique increases the performance of NB and RF classifi- ers. The combination of data sets increases the variability of training samples, providing greater generalization capacity. The stacking technique presents better results than the individual classifications. Accuracy indices greater than 80% are concentrated in the VH polarization channel and are evenly distributed between VGG-16 and VGG-19 CNNs. Fi- nally, the results were compared by the Kruskal-Wallis and Dunn statistical tests, which prove that there is a significant difference between the classification methods, that is, optimizations (parameter settings and combinations of techniques) provide results com- petitive with the literature. Therefore, the research contributes to expanding surveillance methods in the Blue Amazon through artificial intelligence techniques considered state of the art. |
Tipo de Acesso: | Acesso aberto |
URI: | https://www.repositorio.mar.mil.br/handle/ripcmb/846557 |
Tipo: | Dissertação |
Aparece nas coleções: | Telecomunicações: Coleção de Dissertações |
Arquivos associados a este item:
Arquivo | Descrição | Tamanho | Formato | |
---|---|---|---|---|
dissertacao_gabriel.pdf | 12,85 MB | Adobe PDF | Visualizar/Abrir |
Os itens no repositório estão protegidos por copyright, com todos os direitos reservados, salvo quando é indicado o contrário.