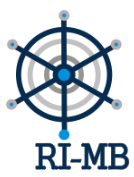
Use este identificador para citar ou linkar para este item:
https://www.repositorio.mar.mil.br/handle/ripcmb/846499
Título: | Assessment of Machine Learning Techniques for Oil Rig Classification in C-Band SAR Images |
Autor(es): | Silva, Fabiano Gabriel da Ramos, Lucas P. Palm, Bruna G. Machado, Renato |
Palavras-chave: | Machine learning Oil rig classification SAR |
Áreas de conhecimento da DGPM: | Sensoriamento remoto |
Data do documento: | 2022 |
Editor: | Remote Sens |
Citação: | da Silva, F.G.; Ramos, L.P.; Palm, B.G.; Machado, R. Assessment of Machine Learning Techniques for Oil Rig Classification in C-Band SAR Images. Remote Sens. 2022, 14, 2966. https://doi.org/10.3390/rs14132966 |
Descrição: | This article aims at performing maritime target classification in SAR images using machine learning (ML) and deep learning (DL) techniques. In particular, the targets of interest are oil platforms and ships located in the Campos Basin, Brazil. Two convolutional neural networks (CNNs), VGG-16 and VGG-19, were used for attribute extraction. The logistic regression (LR), random forest (RF), support vector machine (SVM), k-nearest neighbours (kNN), decision tree (DT), naive Bayes (NB), neural networks (NET), and AdaBoost (ADBST) schemes were considered for classification. The target classification methods were evaluated using polarimetric images obtained from the C-band synthetic aperture radar (SAR) system Sentinel-1. Classifiers are assessed by the accuracy indicator. The LR, SVM, NET, and stacking results indicate better performance, with accuracy ranging from 84.1% to 85.5%. The Kruskal–Wallis test shows a significant difference with the tested classifier, indicating that some classifiers present different accuracy results. The optimizations provide results with more significant accuracy gains, making them competitive with those shown in the literature. There is no exact combination of methods for SAR image classification that will always guarantee the best accuracy. The optimizations performed in this article were for the specific data set of the Campos Basin, and results may change depending on the data set format and the number of images. |
Tipo de Acesso: | Acesso aberto |
URI: | https://www.repositorio.mar.mil.br/handle/ripcmb/846499 |
Tipo: | Artigo científico |
Aparece nas coleções: | Hidrografia e Navegação: Coleção de Artigos |
Arquivos associados a este item:
Arquivo | Descrição | Tamanho | Formato | |
---|---|---|---|---|
3_artigo_remotesensing-14-02966-v2-1.pdf | 9,76 MB | Adobe PDF | Visualizar/Abrir |
Os itens no repositório estão protegidos por copyright, com todos os direitos reservados, salvo quando é indicado o contrário.